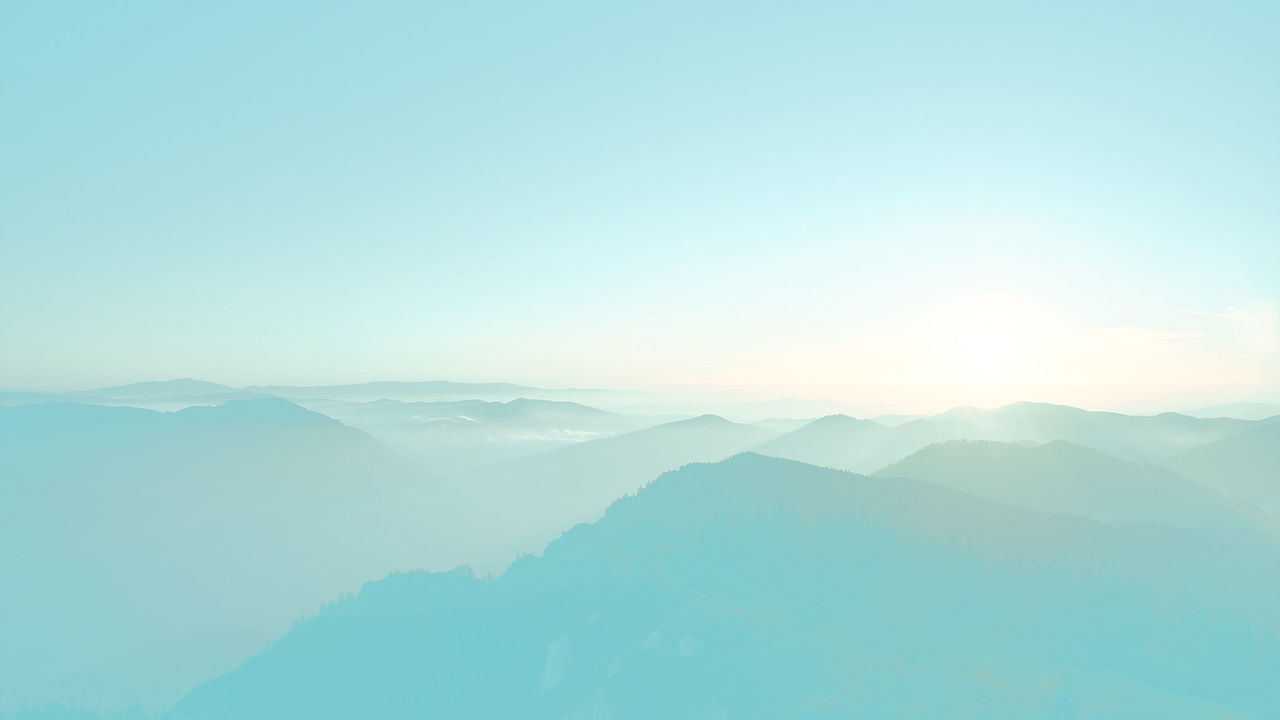
Automating SPECIES IDENTIFICATIONS
Identifying species is a long-term challenge in biodiversity science, often requiring expertise garnered through years of study. That expert knowledge, however, is often based on visual or auditory recognition, such that new machine-learning approaches may be able to "learn" that expertise and make the identification process much less cumbersome.
​
The Peterson Lab got involved in this very exciting possibility via funding from the KU Office of the Provost, which funded the "Virtual Vector Lab." VVL was (is) a collaboration among the Biodiversity Institute, the Information and Telecommunication Technology Center, and the Spencer Art Museum, and focused on automating identifications of the relatively large bugs that transmit Chagas disease ("kissing bugs," as they are called). That early work resulted in a series of publications on automating identification of Chagas bugs, and demonstrated the promise of the approach.
​
A collaboration between Peterson Lab graduate Ali Khalighifar and ITTC's Ed Komp led to exploration of a new technology, that of deep-learning algorithms, in particular TensorFlow. Ali went on to explore the application of this technology to a number of systems, listed below. Ali's doctoral dissertation defense, which is a nice presentation of much of this work, can be viewed here.
Philippine frogs
Frog diversity in the Philippines is focused in the genus Platymantis, which is speciose and can present difficult identification challenges. Here, Ali used their call notes, and subjected them to deep-learning algorithms, and found excellent discrimination and identification ability.
Kansas mosquitoes
Mosquitoes are significant disease vectors, and some particular species represent particular concerns as far as disease transmission. This study focused on identifying the mosquitoes of Lawrence, Kansas, by their wing "whine" noise, and had the objective of assessing whether automated systems would be able to detect the arrival of invasive mosquito species of particular concern. Paper is here.
North American ticks
This is work in progress, but Ali and Susan Little (Oklahoma State University) and Marlon Cobos are working to automate identification of North American ticks based on dorsal views taken with cell phone cameras. Initial test results based on high-quality images showed immense promise, and this work is in the latter stages of analysis and development for publication.